Microcanonical Langevin Monte Carlo#
This is an algorithm based on https://arxiv.org/abs/2212.08549 ([RDLSS23], [RS23]). A website with detailed information about the algorithm can be found here.
The original derivation comes from thinking about the microcanonical ensemble (a concept from statistical mechanics), but the upshot is that we integrate the following SDE:
where \(u\) is an auxilliary variable, \(S(x)\) is the negative log PDF of the distribution from which we are sampling and the last term describes spherically symmetric noise. After \(u\) is marginalized out, this converges to the target PDF, \(p(x) \propto e^{-S(x)}\).
How to run MCLMC in BlackJax#
It is very important to use the tuning algorithm provided, which controls the step size of the integrator and also \(L\), a parameter related to \(\eta\) above.
An example is given below, of a 1000 dim Gaussian (of which 2 dimensions are plotted).
Show code cell content
import matplotlib.pyplot as plt
plt.rcParams["axes.spines.right"] = False
plt.rcParams["axes.spines.top"] = False
plt.rcParams["font.size"] = 19
import jax
from datetime import date
rng_key = jax.random.key(int(date.today().strftime("%Y%m%d")))
import blackjax
import numpy as np
import jax.numpy as jnp
def run_mclmc(logdensity_fn, num_steps, initial_position, key, transform):
init_key, tune_key, run_key = jax.random.split(key, 3)
# create an initial state for the sampler
initial_state = blackjax.mcmc.mclmc.init(
position=initial_position, logdensity_fn=logdensity_fn, rng_key=init_key
)
# build the kernel
kernel = blackjax.mcmc.mclmc.build_kernel(
logdensity_fn=logdensity_fn,
integrator=blackjax.mcmc.integrators.isokinetic_mclachlan,
)
# find values for L and step_size
(
blackjax_state_after_tuning,
blackjax_mclmc_sampler_params,
) = blackjax.mclmc_find_L_and_step_size(
mclmc_kernel=kernel,
num_steps=num_steps,
state=initial_state,
rng_key=tune_key,
)
# use the quick wrapper to build a new kernel with the tuned parameters
sampling_alg = blackjax.mclmc(
logdensity_fn,
L=blackjax_mclmc_sampler_params.L,
step_size=blackjax_mclmc_sampler_params.step_size,
)
# run the sampler
_, samples, _ = blackjax.util.run_inference_algorithm(
rng_key=run_key,
initial_state_or_position=blackjax_state_after_tuning,
inference_algorithm=sampling_alg,
num_steps=num_steps,
transform=transform,
progress_bar=True,
)
return samples
# run the algorithm on a high dimensional gaussian, and show two of the dimensions
sample_key, rng_key = jax.random.split(rng_key)
samples = run_mclmc(
logdensity_fn=lambda x: -0.5 * jnp.sum(jnp.square(x)),
num_steps=1000,
initial_position=jnp.ones((1000,)),
key=sample_key,
transform=lambda x: x.position[:2],
)
samples.mean()
Array(0.0127253, dtype=float32)
plt.scatter(x=samples[:, 0], y=samples[:, 1], alpha=0.1)
plt.axis("equal")
plt.title("Scatter Plot of Samples")
Text(0.5, 1.0, 'Scatter Plot of Samples')
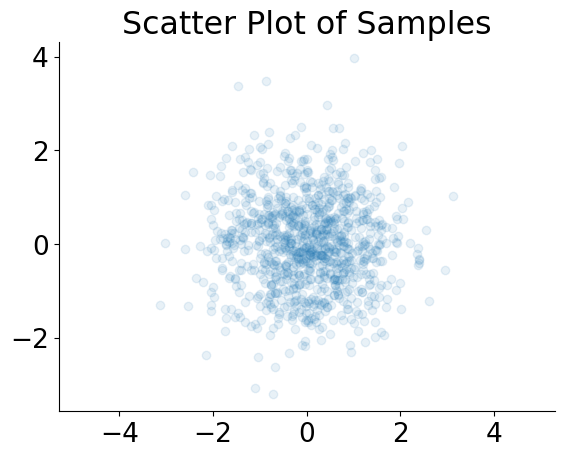
Second example: Stochastic Volatility#
This is ported from Jakob Robnik’s example notebook
import matplotlib.dates as mdates
from numpyro.examples.datasets import SP500, load_dataset
from numpyro.distributions import StudentT
# get the data
_, fetch = load_dataset(SP500, shuffle=False)
SP500_dates, SP500_returns = fetch()
# figure setup
_, ax = plt.subplots(figsize=(12, 5))
ax.spines["right"].set_visible(False) # remove the upper and the right axis lines
ax.spines["top"].set_visible(False)
ax.xaxis.set_major_locator(mdates.YearLocator()) # dates on the xaxis
ax.xaxis.set_major_formatter(mdates.DateFormatter("%Y"))
ax.xaxis.set_minor_locator(mdates.MonthLocator())
# plot data
dates = mdates.num2date(mdates.datestr2num(SP500_dates))
ax.plot(dates, SP500_returns, ".", markersize=3, color="steelblue")
ax.set_xlabel("time")
ax.set_ylabel("S&P500 returns")
/opt/hostedtoolcache/Python/3.9.18/x64/lib/python3.9/site-packages/tqdm/auto.py:21: TqdmWarning: IProgress not found. Please update jupyter and ipywidgets. See https://ipywidgets.readthedocs.io/en/stable/user_install.html
from .autonotebook import tqdm as notebook_tqdm
Downloading - https://d2hg8soec8ck9v.cloudfront.net/datasets/SP500.csv.
Download complete.
Text(0, 0.5, 'S&P500 returns')
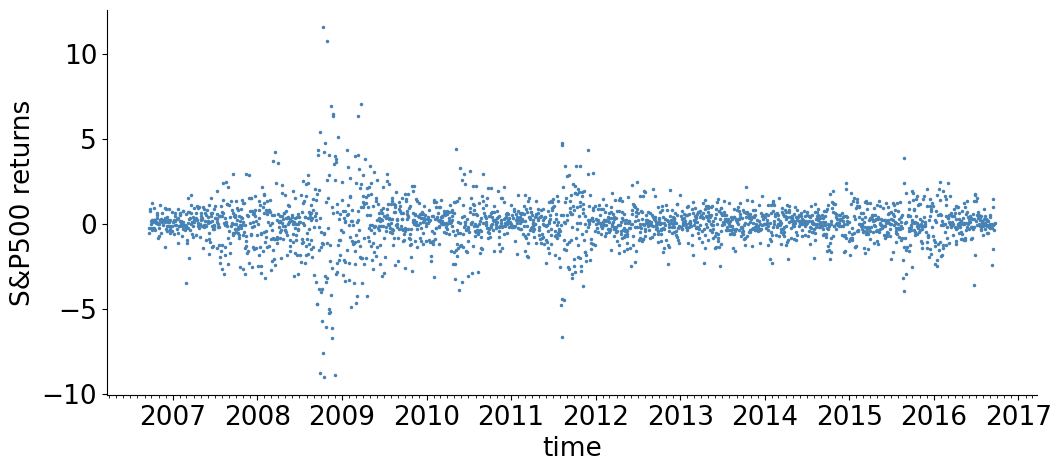
dim = 2429
lambda_sigma, lambda_nu = 50, 0.1
def logp(x):
"""log p of the target distribution"""
sigma = (
jnp.exp(x[-2]) / lambda_sigma
) # we used log-transformation to make x unconstrained
nu = jnp.exp(x[-1]) / lambda_nu
prior2 = (jnp.exp(x[-2]) - x[-2]) + (
jnp.exp(x[-1]) - x[-1]
) # - log prior(sigma, nu)
prior1 = (dim - 2) * jnp.log(sigma) + 0.5 * (
jnp.square(x[0]) + jnp.sum(jnp.square(x[1:-2] - x[:-3]))
) / jnp.square(
sigma
) # - log prior(R)
lik = -jnp.sum(
StudentT(df=nu, scale=jnp.exp(x[:-2])).log_prob(SP500_returns)
) # - log likelihood
return -(lik + prior1 + prior2)
def transform(x):
"""transform x back to the parameters R, sigma and nu (taking the exponent)"""
Rn = jnp.exp(x[:-2])
sigma = jnp.exp(x[-2]) / lambda_sigma
nu = jnp.exp(x[-1]) / lambda_nu
return jnp.concatenate((Rn, jnp.array([sigma, nu])))
def prior_draw(key):
"""draws x from the prior"""
key_walk, key_exp1, key_exp2 = jax.random.split(key, 3)
sigma = (
jax.random.exponential(key_exp1) / lambda_sigma
) # sigma is drawn from the exponential distribution
def step(track, useless): # one step of the gaussian random walk
randkey, subkey = jax.random.split(track[1])
x = (
jax.random.normal(subkey, shape=track[0].shape, dtype=track[0].dtype)
+ track[0]
)
return (x, randkey), x
x = jnp.empty(dim)
x = x.at[:-2].set(
jax.lax.scan(step, init=(0.0, key_walk), xs=None, length=dim - 2)[1] * sigma
) # = log R_n are drawn as a Gaussian random walk realization
x = x.at[-2].set(
jnp.log(sigma * lambda_sigma)
) # sigma ~ exponential distribution(lambda_sigma)
x = x.at[-1].set(
jnp.log(jax.random.exponential(key_exp2))
) # nu ~ exponential distribution(lambda_nu)
return x
key1, key2, rng_key = jax.random.split(rng_key, 3)
samples = run_mclmc(
logdensity_fn=logp,
num_steps=10000,
initial_position=prior_draw(key1),
key=key2,
transform=lambda x: x,
)
samples = transform(samples.position)
R = np.array(samples)[:, :-2] # remove sigma and nu parameters
R = np.sort(R, axis=0) # sort samples for each R_n
num_samples = len(R)
lower_quartile, median, upper_quartile = (
R[num_samples // 4, :],
R[num_samples // 2, :],
R[3 * num_samples // 4, :],
)
# figure setup
_, ax = plt.subplots(figsize=(12, 5))
ax.spines["right"].set_visible(False) # remove the upper and the right axis lines
ax.spines["top"].set_visible(False)
ax.xaxis.set_major_locator(mdates.YearLocator()) # dates on the xaxis
ax.xaxis.set_major_formatter(mdates.DateFormatter("%Y"))
ax.xaxis.set_minor_locator(mdates.MonthLocator())
# plot data
ax.plot(dates, SP500_returns, ".", markersize=3, color="steelblue")
ax.plot(
[], [], ".", markersize=10, color="steelblue", alpha=0.5, label="data"
) # larger markersize for the legend
ax.set_xlabel("time")
ax.set_ylabel("S&P500 returns")
# plot posterior
ax.plot(dates, median, color="navy", label="volatility posterior")
ax.fill_between(dates, lower_quartile, upper_quartile, color="navy", alpha=0.5)
ax.legend()
<matplotlib.legend.Legend at 0x7f57e5c5a160>
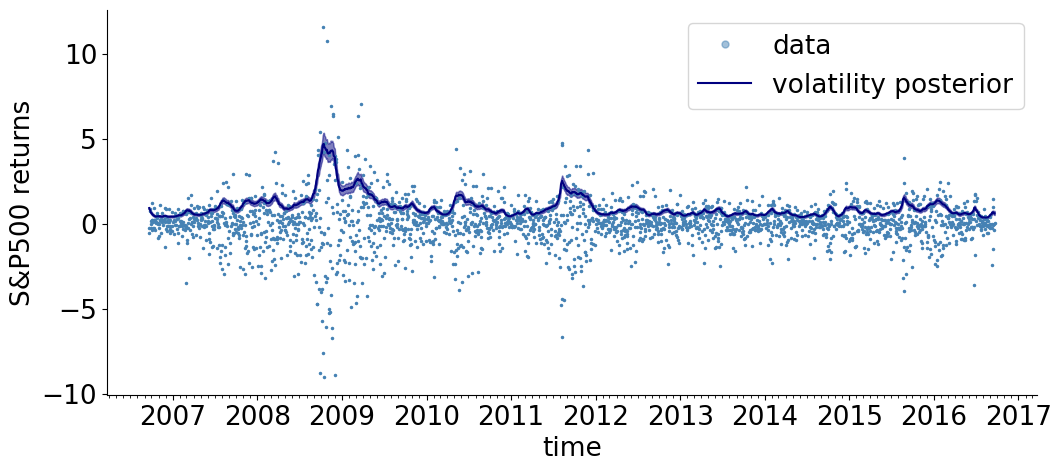